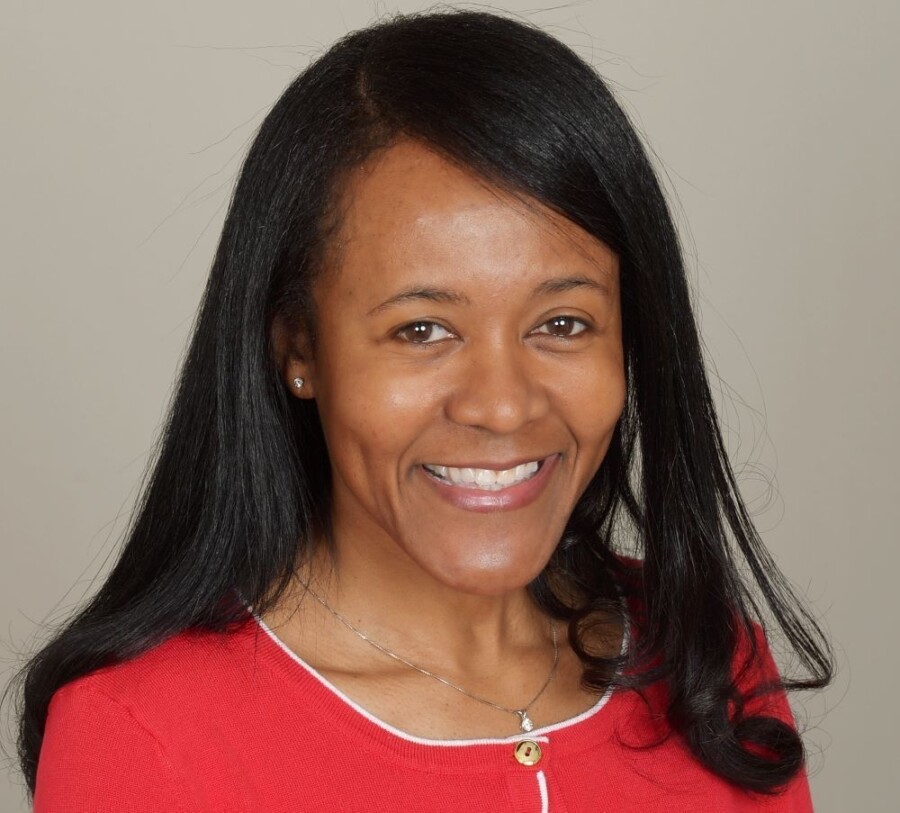
The short answer to this question is: It depends on where they are in their data quality journey prior to the start of the AI project.
One of the first realities that every leader faces when embarking on an AI initiative is data quality. They realize that their data is not as “clean” as they would have hoped. Despite the business systems having certain controls and guidelines to prevent missed data fields or incorrect data formats, they still find so many inconsistencies that prevent the algorithms from yielding meaningful and accurate results. AI project managers learn quickly that an inconsistent data set is just as harmful as missing data.
From data accuracy, consistency, completeness, timeliness, and relevance, each aspect of data quality reveals that significant work is needed to address these aspects before the AI programs can launch successfully.
One company recognized early in the initiative that it faced data timeliness issues. The company learned that data freshness impacted meaningful algorithm outcomes.
In another case, data consistency was the issue, and if the data sets were not corrected, then the algorithm results were skewed in the wrong direction or yielded incorrect insights.
Another case required three years of incorrect historical data review and clean-up before the algorithm patterns and correlations could be trusted.
Exploring the root causes of their data challenges, they went back to the principle concepts based on established data quality standards: “Garbage in” data means “garbage out.”
- Over time, the data quality standards had not evolved, and some key elements to support strong governance were not in place.
- Decisions to introduce data quality tools were deferred due to cost-cutting pressures.
- There were no data quality teams in place to measure and monitor data quality and data-related business processes.
- There was no plan to educate the user community on why data quality mattered.
It wasn’t just one thing; it was many things that led to their data quality situation.
With this new awareness and enlightenment, driven by the desire to take advantage of business AI capabilities, there was a renewed focus on getting data quality initiatives back on track.
Many of our business and IT leaders have found new support and allies to drive investments in data quality efforts. The energy and excitement about the promise of AI to increase productivity and drive efficiency has elevated the interest in addressing the root causes impacting data quality. Leaders are now gaining the resources to put rigorous and structured data governance frameworks and tools in place to help drive sustainable change.
As you consider your journey and strategy to take advantage of AI use cases for your business, remember to take a step back and evaluate your data plan. Think about it by going back to the basics and considering your governance model, your team, your continuous education efforts, and your key performance metrics. How are you doing? Is your team ready? And even more importantly, Is your data ready?
Kimberley Reid is VP of Digital Enterprise, SAP Solutions for Hitachi Vantara. She continues her people focused commentaries for ASUG Executive Exchange. Reid draws on everyday technology, project, people, and leadership experiences of her career. She plans a forthcoming book based on these experiences and reflections.