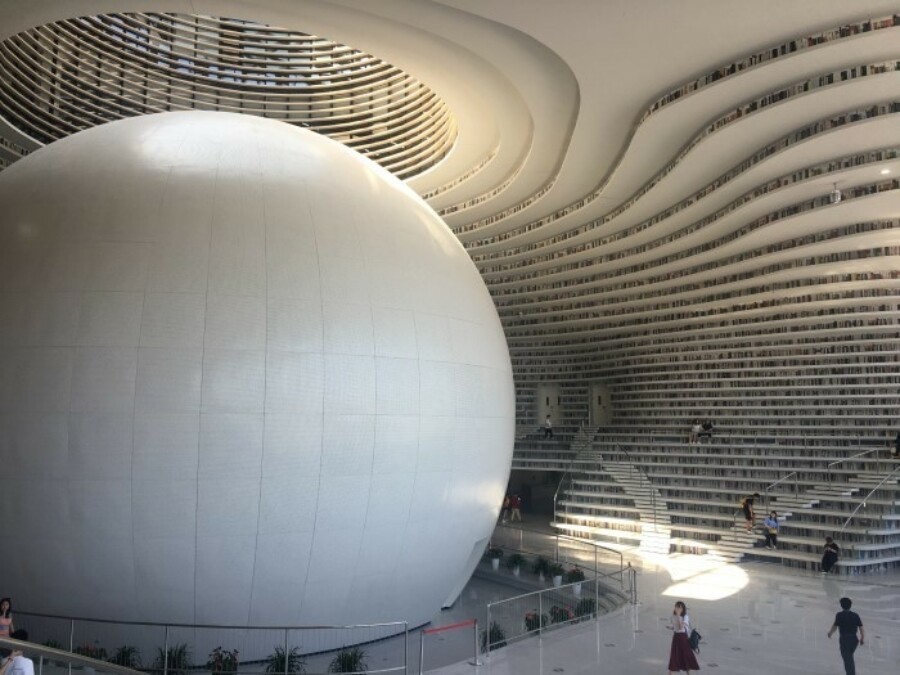
Despite its surging popularity, machine learning isn’t new. It’s been around for more than 50 years, first introduced when a neurophysiologist and mathematician co-wrote a paper on how human neurons might work. To illustrate the theory, they modeled a network with electrical circuits, and soon after, computer scientists began applying the idea to their work.
Today, machine learning certainly has evolved. Companies use machine learning to tap into big data to make forecasts that will drive their businesses forward in areas such as product development, customer satisfaction, and employee recruitment or retention, just to name a few.
Simply stated, machine learning allows an organization to conduct business more intelligently. We sat down with Markus Noga, senior vice president of SAP Leonardo Machine Learning, to better understand how it can be used and how it will continue to evolve.
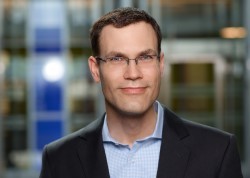
Sharon: What are some of the most innovative uses of machine learning you’ve seen among SAP customers? Which industries are using the technology well?
Markus: It’s great when our customers bring this technology into their core business. An example is Mapal—a German provider of machine tools and precision equipment. Mapal was struggling with managing material, supplier, and logistics information, and its IT team used SAP Leonardo IoT and SAP Cloud Platform to extract information about materials, tools, equipment, supply chain, and logistics from all the paper and PDF documents. By making everything digitally available, the company was able to transform the material and tool handling with more than 20% efficiencies in the processes.
Another example is SFR—a major French telecon operator—that uses chatbots and conversational agents from SAP to serve millions of customers in its daily inquiries. It’s able to provide 24/7 availability to answer questions and provide customer support.
And Daikin—a Japanese manufacturer of air conditioning units—is leveraging SAP Leonardo Machine Learning services to manage installation processes with greater efficiency, improved quality control, and reduced costs.
Sharon: Why is now the right time for companies to invest in machine learning? Particularly, why should future SAP S/4HANA customers look at this now instead of after their implementation?
Markus: Now is absolutely the right time because we are progressing on two main fronts. Number one, the volume of embedded intelligence in SAP’s business applications is growing leaps and bounds. It’s in more than 100 use cases embedded in SAP S/4HANA, SAP C/4HANA, SAP Ariba, SAP Concur, and in all of SAP’s line of business solutions that help finance, procurement, and HR run better.
The second reason is that we have just released a major upgrade to the tooling that’s underneath, enabling your IT teams and partners to transform your core business with solutions.
For example, SAP Intelligent Robotic Process Automation (IRPA) is helping drive automation. SAP Data Intelligence is helping to build models and learn from data. SAP Conversational AI and SAP CoPilot continue to transform customer interactions and customer service into actions on a global scale.
Sharon: What is the biggest myth about machine learning that needs to be debunked?
Markus: I think the biggest one is probably that machine learning and artificial intelligence (AI) are like fairy dust that you sprinkle over a problem and the problem goes away. It is a very powerful technology, but it’s not fairy dust. Applying this requires good engineering, good data science, and a careful eye for the limitations of the technology. And I think that process, particularly working with customers who come in with a fairy dust expectation, is one that requires very careful expectation management.
Sharon: At TechEd last year, SAP announced that it wanted to deliver a unified experience across all SAP technologies, simplifying the deployment of machine learning models into production. Where is SAP now in terms of delivering on that?
Markus: We’ve been pretty good at delivering on that promise. First, with delivering a unified experience, we’ve brought together the SAP Data Hub and SAP Leonardo Machine Learning Foundation to introduce SAP Data Intelligence. It has one lifecycle going from data to machine learning to embedding intelligence in applications, making it uniform with one developer experience, one deployment, one management, and one operations experience.
The second point is on the recent acquisition of Contextor, which we’ve integrated with the organic development of the cloud and released SAP IRPA.
The third point I’ll make, and I discussed this earlier, is that we’ve been very busy merging the technology underneath SAP CoPilot Assistance with our conversational AI. And we’re not stopping there. We’ve also made the commercial consumption of these technologies a lot easier by making them available under Cloud Platform Enterprise Agreement. So, a new product launching, like SAP’s IRPA for example, has hundreds of customers already subscribed from the get-go because we are under Cloud Platform Enterprise Agreement with all our intelligent platforms.
Sharon: Data is key to the accuracy and stability of machine learning. What is SAP doing to help customers make sure their data is clean, accurate, and useful before they embark on a machine learning project?
Markus: That’s a very important question because the models we train and the algorithms we build only can be as good as the data that we’re working with. And any biases or imbalances in the data will show in the performance of that algorithm.
There are two main points I want to make. The first one is for embedded intelligence. We work with a cross section of data from lead customers and co-innovation customers to make sure the models we build are fit-for-purpose in a variety of businesses and geographies.
For example, when we started out matching financial transactions, we learned that a model that works in central Europe doesn’t necessarily work well in the Nordics or in Asia-Pacific. And we’ve had to substantially expand these models to make sure they’re fit-for-purpose globally by training models with data from all these spaces. So, customers get an embedded model that is proven to work well across geographies.
The second point is on tooling. SAP Data Intelligence starts out with connection to the data source, whether SAP or non-SAP systems. And then our data preparation tooling, where you can, in the browser, work in a spreadsheet to correct data and put in your adaptations, is just as available in that tool and one development environment like all the other steps of the data science value chain. So, we’re giving our users the tools to also prepare their own models with great diligence and care.
Sharon: How is machine learning or AI finding its way into the analytics side?
Markus: SAP Analytics Cloud has done a few things with machine learning, which should already be visible to end-users today. Things like the speech-based query, or natural language processing.
We’ll be working together to also include more capabilities which go beyond the casual user. So, everything that you do—every model, every pipeline that you build—in SAP Data Intelligence can work natively on the SAP HANA database and can export into an SAP Analytics Cloud.
When your data science team has built that analysis on the average user, it has a seamless way to publish and integrate into its analytics environment.
Sharon: Can you describe the Next-Generation Support from SAP that leverages machine learning and AI to improve customer experience?
Markus: We started by trying this out internally with projects from our global IT team, and they built things like chatbots for IT support. They called it AyTee, and it’s live and in production on SAP’s corporate portal for the 100,000 employees needing IT support.
We built it on top of SAP’s own conversational AI platform so that somebody encountering problems on whatever system—Outlook, Windows, or whatever—can get rapid-fire support just a message away. And that’s getting good adoption.
We’ve also worked with customers like CC Energy to help support its business in doing external customer service and support. CC Energy gets more than 300,000 customer inquiries every year. And we’re helping the company with SAP Service Ticket Intelligence to better understand and manage the inquires arriving via email.
Our next step is now working with the SAP Global Services and Support team to turn all this intelligence into SAP’s own customer service and support.
The SAP Digital Business Services (DBS) team has been incorporating machine learning and AI in the way it provides customer service and support with the same technologies and tools. Customers should already be seeing this now in accelerated response times and increased relevance of the feedback that’s coming back.
Sharon: What is next for SAP machine learning capabilities? What do you see this offering looking like one to two years from now?
Markus: If we’re starting out near-term, what customers should be expecting is for embedded machine learning to become even more pervasive in all the apps. We have 100 embedded capabilities across the apps portfolio right now. By the end of the year it’s going to be 70 more.
One example is with SAP S/4HANA, where we’re now shipping automated financial close content. RPA bots come standard with the solution for things like automated journal entries. There are six capabilities like this already shipping with SAP S/4HANA today. By the end of the year we’re going to see around 50 intelligent bots already shipping with SAP S/4HANA as part of the standard in finance, in procurement, in manufacturing, and one or two other domains. So, this story about accelerating how we embed intelligence in the apps is one where we’re set to continue.
You also can now expect SAP’s Data Intelligence to significantly deepen and accelerate our support for every machine-learning technology out there. We’re already open in the sense of having more SAP technologies and the best of the open source world. Expect this to broaden and extend. Also, in the sense of connectivity to systems, which we enable to interface with, you can expect SAP CoPilot to completely re-platform on conversational AI, on making that one technology the foundation for intelligent bots that’s becoming pervasive.
And last, but not least, expect us to make significant progress with IRPA, enabling our customers in the here and now to take advantage of intelligent technologies on their roadmap to SAP S/4HANA and the intelligent enterprise.
Interested in learning more about machine learning and what it means for your data strategy? Register for the “How SAP Leonardo Machine Learning Supports Finance Cash Application,” webcast available on demand.