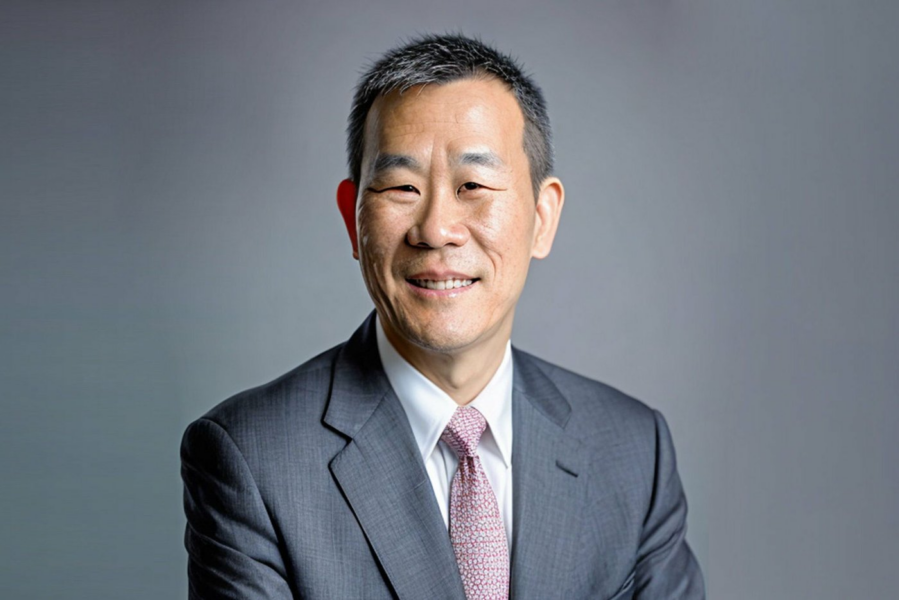
In his position as Global Head of Artificial Intelligence (AI) at SAP, Dr. Walter Sun is working to develop and deploy enterprise AI technology to solve for customers’ most pressing business issues.
Having spent 18 years previously working at Microsoft, where he held senior scientific and product development positions, Sun is responsible for leading the AI strategy for SAP and has been an influential voice in closing the distance between the generative-AI hype cycles and real business results for the SAP global user base.
Ahead of ASUG Tech Connect, where Sun will discuss exploring artificial intelligence for real-world results and help to deliver the conference’s day-three keynote focused on uniting customers, partners, and SAP through AI and strategic collaboration, Sun sat down with ASUG to discuss the SAP-Microsoft AI partnership, the importance of deep and bi-directional integration between digital copilot Joule and Microsoft Copilot, how WalkMe will augment Joule’s governance capabilities, and what role SAP will play in upskilling customers to get the most value out of AI technologies.
This interview has been edited and condensed.
At a high level, how would you articulate the SAP Business AI strategy to our readers?
At SAP, our strategy for delivering business AI breaks down into three core pillars.
The first is Joule, our digital copilot, which connects SAP applications and also enables extension by customer-built apps—so across everything for business users’ and developers’ apps. Secondly, we embed AI directly in the application layer. For example, in SAP SuccessFactors, users can use generative AI to automate the creation of job descriptions when someone is looking to fill a role. And thirdly, we’re focused on creating capabilities for developers. We offer a wealth of developer tools on the SAP Business Technology Platform. A key example is our Generative AI Hub, which allows both internal and external developers to access a variety of large language models.
We currently support 25 of them. We have an “abstraction layer,” where we have customers give us a use case, and we can find the right large language model for them, which is the one that gives them the best response — the best bang for the buck, if you will.
In other words, if you told me you wanted to create a QA-bot engine, and I told you these five large language models will all will give you the same performance, you can say, “Give me the cheapest one,” which is both less cost for you and your company, as well as better in terms of the sustainability footprint, with the least amount of compute necessary. That’s how we think about our multi-vendor strategy. We want to help the business user find the best large language model for them.
Given your background at Microsoft, what excites you about the partnership announced at SAP Sapphire, through which Joule will be integrated with Microsoft Copilot to establish a more open-ended, partner-centric evolution of business AI?
At SAP, we believe in partnerships to help our end users benefit the most. SAP powers the vast majority of Fortune 500 companies; 99 of the 100 biggest companies use SAP. We’re the leader in business applications. At the same time, many of these customers use Microsoft's Office Productivity Suite. As you can see, and as you observed, there are immediate synergies there.
Instead of a user working through two different copilots, which don’t speak to one another, we have a partnership where, if you have Microsoft Copilot and you have a business application-specific question that SAP can answer, it can invoke Joule and say, “Hey, Joule, can you handle this one?” Likewise, in Joule, if you have questions about Microsoft Graph, or other topics related to the Microsoft Copilot, we can ask the Microsoft Copilot for that information. Given that we have a lot of mutual customers, this makes it easier for people who run both not to have to jump from one copilot to the other.
Without personifying copilots, if you consider them as digital assistants, without having to go to two different assistants and ask them questions separately, or go to eight or 20 different assistants in the long run, our vision is that you have partnerships — starting with Microsoft, but also with many other copilots that exist, so that we can make it easy for our business users. We can tell them, “Go to Joule, and everything will be there, from connection to all other business applications that SAP has to extensibility across other business applications in your space.”
In terms of the deep, bi-directional integration that you’re discussing, and in terms of embedding Joule in SAP S/4HANA Cloud, SAP Build, SAP Integration Suite, SAP Ariba, SAP Analytics Cloud, and more, what excites you the most about what this will enable for business users?
What’s exciting to me is that we can help users go across different SAP applications. I’ve demonstrated a Joule copilot engagement in SAP Concur, wherein you can ask it about booking a flight for a business trip, ask it also about extending that trip and using vacation days to do so, have it engage SAP SuccessFactors to determine if I have vacation days left, then come back into SAP Concur and add two vacation days to the trip. That’s one example.
The next level involves a collaborative, multi-agent framework. If you’re refurbishing a building, how do you approach that innovation? Normally, you’d contact planning agents and pricing agents, but you can alert Joule to your plan, and various agents will act on your behalf, pulling the relevant information from your databases and negotiating with one another. Imagine an agent for SAP S/4HANA looking at the supply chain, an agent for finances, and an agent for accounts receivable, all coordinating behind the scenes. Today, in a Microsoft Teams meeting with multiple colleagues, you might ask somebody to find you the SOW template; tomorrow, an AI agent with pricing skills will determine the cost to buy 100 laptops, and then a separate SOW agent will obtain your approval and write the SOW for you.
Making life easier for business users, we’ll have these digital agents in the background doing that type of work, and if what you need doesn’t fit within the SAP ecosystem, we have these copilot connections to Microsoft, where you can get additional information from your Microsoft Office Graph and extend the space. That extensibility, both through a copilot like Joule at the top level and the extensibility of other people building mini-agents, can allow us to do much more with what we have.
SAP recently completed its acquisition of WalkMe, which was announced at SAP Sapphire earlier this year. Tell me more about the motivation behind that and where you see WalkMe integrating with Joule and this overarching vision for business AI.
WalkMe is a leading digital adoption platform provider; acquiring them helps SAP increase its focus on the success of the business software user. Their technology gives users enhanced guidance and automation features that allow them to perform workflows smoothly across all different applications, including third-party applications.
Users are able to master very complex digital processes with simple on-screen guidance and analytics. It increases usage of those applications and drives value creation to our customers. We feel like the acquisition complements our SAP Business Transformation Management portfolio. It adds a strong people component to our existing business transformation, which has thus far covered the process, applications, and data dimensions. There are those three dimensions that we had; this people component completes the picture.
Having SAP Signavio, SAP LeanIX, SAP Business Technology Platform, and now WalkMe working together, we can help our customers perform a digital transformation journey even faster and more effectively. Of course, WalkMe will help to enhance the productivity of our digital AI assistant; it can overlay web, mobile, and desktop apps, including SAP and non-SAP systems, without integrating online software. By combining WalkMe’s adoption capabilities with SAP’s Joule copilot, SAP will be able to offer better AI assistance in the user experience.
Can you expand on those adoption capabilities that SAP unlocks through combining WalkMe with Joule? From a UX perspective, this could provide an intuitive path into systems for SAP users, which benefits onboarding processes and also relates to the reskilling and upskilling initiatives that SAP is emphasizing both internally and for customers.
It’s a good question you’re asking about adoption. The generative AI space is moving so quickly, and applications add new features so quickly, that the average business user can be overwhelmed by what's available. In Joule, natively, we really want to make it as easy as possible to actually communicate with applications without having to learn their manuals,
A year ago, when I joined SAP, SuccessFactors was new to me; to figure out where I could open a job description took work. Going into SAP S/4HANA and looking at ERP tools, it took work. Now, a new hire can use natural-language prompts and say, “I want to know how to how many vacation days I get a year. What's the company policy on business travel? Who are our ten biggest supply chain providers?” That information can be gathered with natural language.
WalkMe goes even further where it actually provides the automated features and workflows upfront; you have guidance. In addition to having the existing application software, a digital adoption platform helps people see how you use different processes. There’s on-screen guidance and analytics saying, “This is what you need to do.” Almost like a human guide, it says, “This a new application you never use. These are some tools in terms of how you can do better.” Based on people's activities in the SAP or non-SAP applications, WalkMe can overlay guidance and provide further assistance, on top of what Joule does, in terms of making it easier for any business user to use technology.
So much of the promise of business AI is simplification of processes for business users. At the same time, as an emerging technology, AI presents opportunities for people to reskill and upskill to effectively navigate AI-embedded applications. With SAP in the midst of its own reskilling initiatives, what capabilities can SAP users build in their organizations to be able to most effectively harness business AI?
SAP has long invested in training measures, based on the direction of the tech industry, to stimulate our growth and keep up with market demand. We hosted a bunch of AI Days in 2023 internally, for our employees to quickly learn and upskill themselves in generative AI. As the number of skills expected for each of our roles increases, and as skills turn over and these roles accelerate, SAP is on its way to becoming a skills-led organization.
Today, we’re enhancing, building, and shifting our learning programs to follow the trends of generative AI; looking at our enterprise cloud services; and looking at all the different necessary skills that we think are necessary for the AI space. Now, we have AI training courses, not only on machine learning and AI but generative AI technology as well.
Across industries, the technology out there democratizes the ability for our customers to do this as well. SAP is building “ABAP AI tools,” or development tools for our ABAP domain-specific language. Think about that as democratizing the ability to write code, because there’s a subset of users in the world that know ABAP; now, in natural language, you can open up the door to how many people can actually write ABAP code with machine assistance. The same is true of the Joule copilot’s tools for using natural-language commands to complete actions. It’s not just pro-code; now, you’ve got low-code capabilities for writing scripts or flow charts, and no-code capabilities via natural language. People are able to do more.
In terms of upskilling, what we need to understand now is how to speak to machines.
With our generative AI hub, SAP is including “prompt management” tools. That’s a fancy way of saying “ways to speak to machines better.” You have horse whisperers that can work with horses, you have nannies that work well with children, and you can have computer whisperers with these prompt engineers. How do you actually ask for something most appropriately to help get the best answer?
I’ve used an analogy that these large language models are almost like children that know a lot of information but don’t have a lot of extra reasoning, so you actually provide specific instructions: “Hey, can you tell me what the hours of this restaurant are, and can I make it to the restaurant if I drive from this location to this location at this time period?” And if the answer is “yes,” that's what you want, rather than the machine saying, “this restaurant is currently open.”
If you ask if it will still be open when you get there, the machine knows that you’re asking a more specific question: not just, “is it open at this current moment,” but “is it going to be open when I arrive, given that I am 60 minutes away?” All that information is the upskilling: how do you communicate with machines better?
That level of intuition will be a particularly interesting challenge in heavily regulated or highly technical industries, where users will need highly specific questions to hone AI tools to assist them. What role will SAP play in helping its customers to learn those skills?
We’ll help in a few ways. We offer retrieval-augmented generation (RAG) technology, which is basically an index that the customer brings in themselves. If ASUG has a set of HR policy guidelines, you can upload those documents into your tenant, privately. And whenever you want to use a language model, that model can first pull information from the tenant to get information. That’s valuable, for three reasons.
- Specificity: If you ask a question about ASUG, the internet has information, so the large language model knows something about ASUG, but not a lot. You can actually get specific information.
- Privacy: Your company's vacation policy is not going to be available on the internet.
- Temporal nature: If I ask whether the Chicago Cubs won last night, the large language models won’t know, but if it can pull from a document database, it can find the right answer.
We’re helping people index information. We're providing prompt management tools to help people do the best they can with each language model. And each language model is different; it's almost like speaking to different people, if you will. In each case, we want to make sure the prompts are set appropriately.
And we also have prominent checks on the output side to help double-check, to make sure the information is correct. How do we minimize hallucinations? If we give you an input that says, “We’re talking to ASUG, based in Chicago,” and then a large language model writes me a story about ASUG, and it says it’s in Wisconsin, we can check the fact is incorrect, with basic fact-checking capabilities.
We can also leverage other language models and call language models again to double-check; each instance of a language model call is almost like asking a different model. And so, models themselves can help to check information. In regulated industries, in many business cases, you can’t afford to make any errors. Making mistakes during a dinner conversation is not going to cost anybody a billion dollars, but making a mistake in a press meeting or a quarterly release could cost a public company a lot of money. We need to make sure that we have the tools to give our customers the highest confidence possible, in terms of what we're providing them.
There’s a saying: “garbage in, garbage out.” Maybe the positive spin is: “good information in, good information out.” People sometimes have negative experiences with large language models, because they're not giving it the right information. They might either provide wrong context or actually ask something that's outdated: “Who's the leader in sustainability?” If you ask that question to a language model that was trained a year ago; perhaps the answer has changed since then.
One needed to actually provide a new context to help to get the right answer. It’s no fault of the user, because it's a very complicated space, helping customers understand when the language models we're using were last trained, letting them know we need to provide newer information before asking time-sensitive questions. The goal, we hope, is that we can help educate our users on how to best use these models to their benefit.
In infusing Joule with multiple autonomous AI agents, what data structure will be required to ensure that these agents can be genuinely collaborative, and what importance does the “human in the loop” approach play?
For different solutions, the structure could differ per business function, such as HR or Finance. But the good news is that we recently announced an SAP Knowledge Graph which helps bridge the gap across these industries and solutions, like manufacturing versus energy versus retail.
Regardless of our SAP Knowledge Graph's ability to parse different terminology across different functions, having a human confirm and review the outcomes will always be of great importance to ensure the best possible outcome of an AI agent’s response.
With the introduction of SAP Knowledge Graph to ground AI in specific SAP business semantics and interrelationships, how is SAP ensuring that these capabilities truly ensure reliable AI outputs?
Across our solutions, we often have fields which might contain acronyms; to make it more complex, these could even be acronyms from different languages. For example, Artificial Intelligence would be AI in English, but it’s KI in German. As a result, certain fields could be referenced in many different ways. Our SAP Knowledge Graph allows us to both indicate when two seemingly different entities are the same as well as produce connective relationships, which increases the comprehension of the models to a customer's request.
You can imagine if you ask a person a question about disparate topics that, if he or she happened to know both topics well, they could internally bridge between them and produce a more knowledgeable answer. This is what having the SAP Knowledge Graph can do to ensure more reliable generative AI outputs, with less hallucinations.
For more from Walter Sun, don't miss ASUG Tech Connect, where Sun will discuss exploring artificial intelligence for real-world results and help to deliver the conference’s day-three keynote focused on uniting customers, partners, and SAP through AI and strategic collaboration.