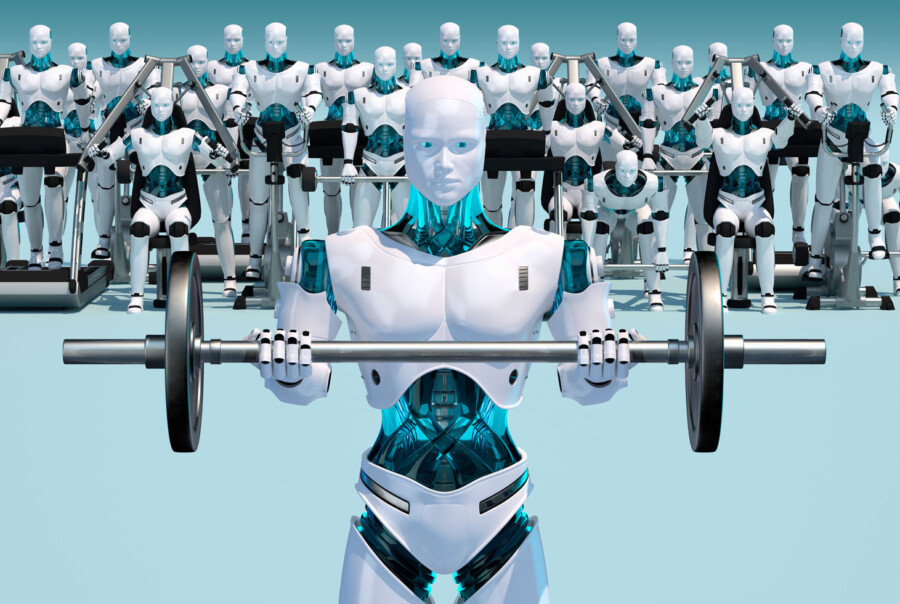
Just shy of 60 years old, machine learning has never looked so good. Exponential data growth, advanced algorithms, and powerful computer processing are enabling the technology to fulfill its ultimate destiny: Identifying profitable opportunities and avoiding unknown risks by evaluating massive volumes of complex data and delivering accurate results in real time.
However, during the Americas’ SAP Users’ Group (ASUG) webcast, “Guide to the Machine Learning Galaxy: How Your ERP Knowledge Enables Value-Driven Intelligent Processes,” Darwin Deano, principal and chief SAP Leonardo officer, and Denise McGuigan, senior manager and Deloitte reimagine platform leader (both from Deloitte Consulting LLP), forewarned that machine learning is only as good as the algorithm, and the algorithm is only as good as the data.
Deano advised, “Data evolves over time. Even though ERP systems provide a strong foundation for identifying opportunities and delivering on the promise of machine learning, it does not factor in information outside the core structure, nor does it move with information as it changes.”
Adding to Deano’s observation, McGuigan noted the importance of understanding data well. “Businesses must know all of the variables and data sets that drive certain decisions. Doing so will reduce the risk of bringing information into the analysis that will only cause noise or false positives within machine learning results,” she shared.
Machine Learning Success Depends on Finding the Right Data “Fit”
Although it’s tempting to jump into machine learning by automating heavily used transactions, McGuigan warned that this view misses the cognitive advantages of machine learning. “Companies have a considerable opportunity to operate with tremendous efficiency and speed,” she said. “They should also consider enabling processes and tasks that free up resources, time, and talent for entering new markets, offering breakthrough products and services, and innovating industry-disruptive business models.”
To successfully execute such an advanced form of machine learning, organizations must ensure that the right data is being applied to the machine learning model. Understanding how each data category impacts the training data helps businesses fine-tune the model to increase prediction accuracy and efficient automation. However, as McGuigan suggested, one of the most common causes of underperforming or inaccurate models can be attributed to an imbalance of data used, commonly referred as biased invariance.
One form of disparity is experienced when the model underfits the training data when assumptions are oversimplified to the point where either the wrong information or too little insight is applied. This condition leads to the inability to capture the relationship between the programmed input examples and the targeted outcomes.
On the flip side, a model can overfit training data when too much information and complexity is used. Even though it performs well with training data, the model cannot accurately evaluate data to deliver the expected outcome. The model only memorizes data, instead of learning from it to generalize how unseen examples should be treated.
Ensuring that the right balance of data is used to optimize the machine learning model is an art of data science. According to McGuigan, businesses must understand the information it has as well as the key inputs and outputs that the model needs. “Once operational needs, stakeholders, and expectations are clearly identified, finding the right data sets becomes easier,” she mentioned. “Businesses need to know ‘why’ the business problem exists first to understand ‘what’ they are trying to solve and the value they will generate.”
It’s also important to remember that this exercise is an iterative process of trial and error. The model may be calibrated well enough at one moment to deliver expected outcomes consistently and predictively; however, as Deano suggested, “what may be overfitting today may not be the same situation six months from now as data evolves.”
For more insights into putting machine intelligence to work for your organization, watch the replay of the ASUG webcast, “Guide to the Machine Learning Galaxy: How Your ERP Knowledge Enables Value-Driven Intelligent Processes,” featuring Deano and McGuigan from Deloitte Consulting, LLP.