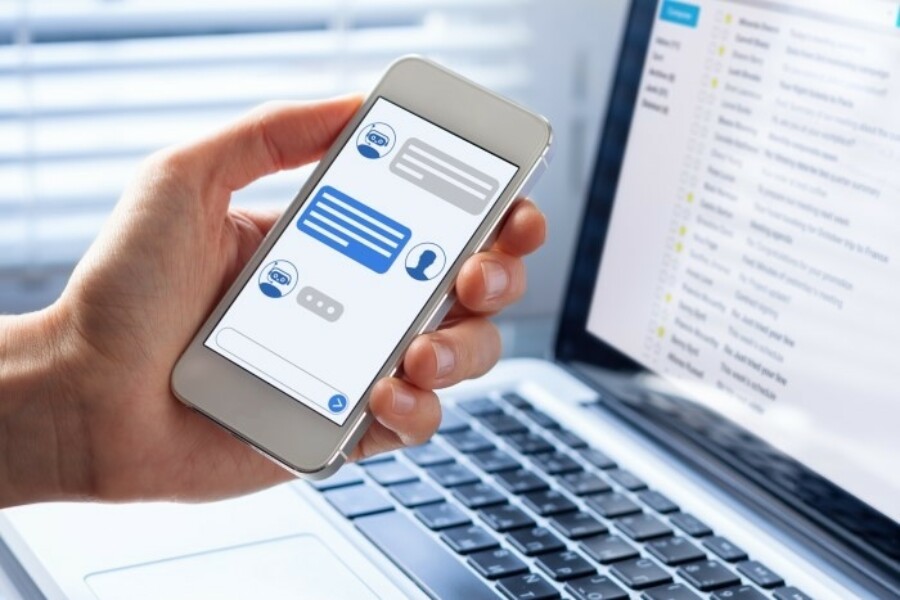
Chatbots and virtual assistants and conversational AI and on and on. We keep hearing about all these emerging technologies, but most of the time these phrases leave us confused as to what it all means, and even more, how to use these emerging technologies within our business operations.
You may have already heard the predictions that suggest by 2020, 70% of customer interactions will involve technology such as chatbots or virtual assistants. ASUG News sat down with Giacomo Gasperini, director, SAP digital commerce, to discuss how SAP is using this technology on its digital channels to improve its customers’ experience, how the technologies fit within the SAP portfolio, and how you can apply them to your own business processes.
Sharon: What is a virtual assistant and what is a chatbot? How are they different? How are they different than artificial intelligence (AI)?
Giacomo: Let’s start with artificial intelligence because it’s an umbrella term that we use to describe machines that mimic those prodigy functions that we normally associate with human minds, such as learning or problem solving. To break it down further, machine learning mimics how humans learn and conversational AI mimics how humans interact.
Now that we have that information and before I talk about the difference between chatbots and virtual assistants, I need to point out that at the current pace of technology, six months can make a big difference. While we’re trying to define these technologies, someone is already working to create a hybrid between them.
Let’s look at how chatbots and virtual assistants are usually defined. Chatbots are made to recognize keywords. They don’t understand meaning and they lose the context if the conversation is interrupted. Virtual assistants such as Apple’s Siri understand natural language. They understand meaning and can stay on track with the conversation to perform complex tasks like booking an appointment. There’s nothing wrong with these definitions, but there are already clear examples of a hybrid of the two.
SAP Conversational AI, for example, is an end-to-end chatbot platform. It has a strong natural language processing engine that allows the bot to understand the user beyond simple keywords. In fact, it’s able to extract the topic from a conversation, store this topic in memory, and allow the bots to stay on track in the conversation. It also can send the conversation to a specific user with a customized outcome.
So, would we define this as a chatbot or a virtual assistant? To me the line between the two is now a lot more blurred than it was even six months ago. As time goes by, it will blur even more, but add more value to the user experience as a result.
Sharon: What are some practical use cases for virtual assistants and chatbots?
Giacomo: Let’s start first by comparing AI to humans. We know that it’s faster than any human. We know that it’s more scalable and that it will provide quick answers independently from how many interactions it’s handling simultaneously. We also know that it’s consistent, it doesn’t get bored or tired, and it always gives you the best possible answer based on its training.
On the other hand, it has no meaning. Siri, for example, doesn’t really understand that you’re sad when you say you are; it’s just mimicking emotional intelligence. Another point is that although huge steps have been obtained in the field of unsupervised learning, the reality is that the best performance in your algorithm is still achieved by a combination of unsupervised with supervised learning.
Allow me to define unsupervised versus supervised learning. In machine learning, supervised learning is this: I feed to the machine a set of structured data. For example, something with four legs is categorized as an animal and with two legs is categorized as human. As a result, I can feed the machine an image and allow it to deduce whether it’s an animal or human based on statistics and what it has learned. Unsupervised learning is when you have a huge amount of unstructured data, you feed it to the machine, and you ask the machine to find the patterns to get to its own understanding. Both are integral for conversational AI.
Let’s now look at what it won’t do. When people think about machine learning, they think that the chatbot is magically going to improve itself based on user interactions. Maybe we’ll get there in five to seven years, but that isn’t the case today, even for advanced platforms. This is because users are not consistent. There is still a need for humans to monitor and train the machine.
Now, let’s get into practical uses cases for conversational AI. It can really add value in those scenarios where users need to perform a potentially growing set of tasks without having received specific training on how to perform them or even know what these tasks are. For example, when you ask Amazon’s Alexa to play a song or check the weather, you don’t know all the tasks it needs to perform to get that outcome. That kind of technology can be applied in a broad spectrum of industries where a user needs assistance but doesn’t quite know all the necessary asks to get the desired outcome.
The way SAP uses it is by helping its customers on their digital purchase journey. SAP sells a growing portfolio of products online. There are more than 50 products to choose from and it’s growing. So, our challenge is that we want to consistently give SAP customers the best information on all our products and the value they can bring to their enterprises, which would require our agents to be experts on everything. This is not realistic. Instead, conversational AI allows us to understand the context that the user is in and tailor the conversation, guide the customer to select the best product, and assist in the checkout or fulfillment process.
It can then escalate to a human agent when the question requires that extra layer of understanding and, even if no agent is available online, create a ticket so that the customer is always connected. We have structured it to extract business intelligence—topic, product, and issue—from the conversation, and combine that with the intelligence coming from the rest of our interaction channels to find out which areas our customers are struggling with. This way we try to improve their experience. After all, you have a user that is considering you as their business partner, the last thing you want is to create an obstacle when they’re making this choice.
Sharon: How do virtual assistants and chatbots fit into SAP offerings such as SAP S/4HANA, SAP C/4HANA, SAP Ariba, SAP SuccessFactors, or SAP Concur?
Giacomo: Let me give you some examples. Our conversational AI bot can create requests in SAP SuccessFactors for a specific user or provide that user with reports from SAP SuccessFactors on how they invest time in projects. The bot is directly connected to a back-end system and offers specific advanced tasks to the user.
We are also working to integrate it with SAP Cloud Platform, and I predict the next two quarters are going to be very exciting because a lot of these projects are going to advance. Several teams at SAP are currently working on integrating conversational AI with additional solutions.
Sharon: What are the challenges of adopting and using this technology? How should customers address change management? How should they address training?
Giacomo: Let me use the SAP Digital Commerce team’s journey with adopting this technology and the challenges we encountered as an example. There were three main challenges—the user, the team, and the technical side.
By user challenge, I mean that customer behavior is changing and the reality is that many users don’t like to interact with machines. Even smart conversational AI doesn’t really understand the way a human does. It can’t sense frustration or other human emotions and if the conversational AI can’t understand, then it can’t react to it. This can be a challenge when we’re trying to gain the trust of our customers. There are many approaches to this challenge, however, and the one we chose was to put the focus on customer feedback as opposed to efficiency as an indicator of success. The first thing we monitor every day is not how many conversations that bot was able to solve, but rather what was the user feedback on these conversations? And then based on this feedback, we work to improve the user experience.
Efficiency will inevitably come, but it will come as a byproduct to implementing automation through conversational AI. This way, you don’t lose the user in the process. The most complex algorithm to crack is not the one of machine learning, it’s how the user wants to ask the question or wants to receive the answer. That’s what makes it a challenge.
The second challenge is of course the team. If you ask people in your organization who are currently interacting with your users to participate, they may perceive automation as a risk, especially when it comes to conversational AI. In our case, our team is an integral part of a good customer experience. The best result is when you combine AI with human intelligence. So, our agents interact with customers every day and their experiences are gold if we want our customers to trust us to the point of purchasing our offers online. The vision we had for our organization from the beginning was that each of our agents should be able to program and use conversational AI as a tool. We involved the team in creating and expanding our digital virtual assistant. This paid off because the team perceives the bot as an additional team member.
The third challenge, the technical aspect, is of course the part that I’m very passionate about. This goes back to my earlier statement about supervised versus unsupervised learning. Training the natural language processing engine of your conversational AI to a decent level of understanding requires a lot of hours. And there is no shortcut to circumvent this.
Yes, our conversational AI platform allows the user to train an intent to a decent level of understanding with as little as 80 to 100 phrases, which is excellent. However, when you start adding several intents, which by the way are simply commands such as, “download a product” or “complete the checkout,” you need to make sure they don’t overlap. If they do, your bot will be confused, and the customer will be frustrated.
If you prepare for these challenges and work to train your bots, the result is that your customer is happy, and you’ll feel confident to delegate more and more conversations to the bot. That’s where the efficiency and the bottom line comes in. So again, back to the user challenge, you need to constantly monitor feedback and use it to improve your training of the bots. It’s an ongoing cycle, but the payoff is huge once you have a rhythm going and iron out the kinks.
Sharon: So, you’re always then monitoring and improving, aren’t you? Those customer expectations will continue to change, won’t they?
Giacomo: Absolutely, you have touched on exactly the right point. Their expectations change over time and you should always be training your bot just as you would any other team member to handle those expectations. The difference being, the bot can handle around 30% to 50% of your traffic, and that’s where efficiency and the bottom line come as a byproduct.
Sharon: What resources are available to customers when learning how to use and implement virtual assistants and chatbots?
Giacomo: I am not a programmer, nor am I a person that is able to write a line of code. And yet, I was able to use the documentation and the tutorials available on our SAP Conversational AI page. I learned about the solution in February 2018 and I was able to demonstrate the first prototype of our bot in April of the same year to our leadership team. These resources are available to anyone; it’s the democratization of bot building. It brings bot building out of the IT department and directly to the business unit.
Sharon: Any words of advice for ASUG members?
Giacomo: “The choice isn’t between automation and non-automation, it’s between whether you use the technology in a way that creates shared prosperity.” These are the words of Erik Brynjolfsson, director of MIT Initiative on the digital economy, and I believe them fully.
If you focus your project on combining artificial intelligence with your team to work toward what really matters—which is your customer—efficiency and the bottom line will come. It will come as a byproduct of implementing automation.
Also, equally important, think of the business challenge you want to address before looking to automation. Many teams are rushing to implement bots, because everybody’s doing it. That’s the wrong approach. Identify the challenge that can be solved with the assistance and start there.
Interested in learning how to use predictive maintenance and machine learning in the Enterprise Asset Management (EAM) space? Register for our on-demand webcast and learn where to start.