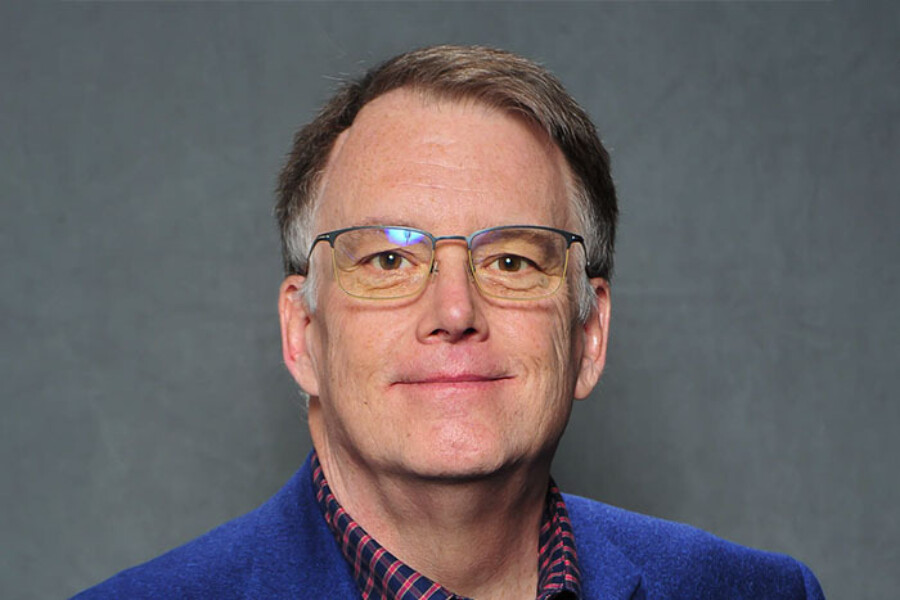
Gartner’s Hype Cycle presents a great model for understanding the maturity lifecycle for new innovations. Following a new idea from the innovation trigger that starts it through the rush of ideation around what it might do, the reality of what it cannot do, and ultimately how it can help technology executives make decisions about when and where to make their investment “bets” on these new ideas.
Unfortunately, when it comes to generative AI, I’m not sure the Hype Cycle model is sufficient.\. AI is hardly a new idea; I asked Google, and it told me the term “artificial intelligence” was first used in the 1950s. The fact that I used an internet search engine to get that factoid clearly demonstrates that at least one AI use case has reached the “plateau of productivity” that Gartner’s Hype Cycle strives for. The problem is that with recent advances in data modeling and hardware capabilities, the use cases for AI have increased significantly, which has, in effect, reset the Hype Cycle for generative AI.
So where does this leave you in understanding the potential role of generative AI in your business? Staying with the Hype Cycle model, many of the use cases you are considering have put you right back at the beginning of the cycle, and that is the crux of what I am hearing from ASUG members as they tackle generative AI.
What are the challenges? I’ll introduce a novel way to answer this question later, but for now, let’s group these challenges into three buckets:
- What can this do for me?
- How do I deploy it?
- What risks does it entail?
There is a fourth bucket: Should I do this? For now, I will set that one aside to focus on the tech and not the ethics of generative AI.
What can this do for me? If you listen to the hype, the answer is “everything.” The reality is less grandiose and relies on how generative AI works. Generative AI models use existing data to identify patterns and use those patterns to infer outcomes based on similar queries. These models are not “creative.” They can make some amazing associations, but those inferences are based on existing patterns. They are not able to create new patterns from a blank page. So if you want to build an AI solution that can predict which new markets your company should enter, think about how you can provide the underlying data so the AI model will “know” this.
How do I deploy it? The details can get very complex, but you basically have two choices on how to implement generative AI: embedded or stand-alone. Embedded AI solutions are becoming available for many enterprise applications you run today, including SAP solutions. When you run these applications, they will leverage AI models to provide insights or decision support, and those decisions and insights will be based on the data available to that model. The main benefit of this choice is that you don’t have to operate and maintain the AI engine in addition to the applications themselves; AI is just a part of a larger application.
Many companies are also deploying generative AI engines as independent solutions and integrating these engines within their own applications. This has the potential to enable AI throughout the enterprise, regardless of whether or not the various solution providers have incorporated AI into their solutions. However, it requires the company to have the skills to support the AI solution in addition to the other solutions in its portfolio.
What risks does it entail? AI creates several risk areas, but rather than going through them, here will use this opportunity to demonstrate AI “in action.” I am going to use a generative AI model—ChatGPT in this case—to explain the risks of using generative AI in business. Here’s what ChatGPT wrote for me when I asked it to write a 400-word article on the business risks of using generative AI:
Title: Navigating the Business Risks of Generative AI: A Prudent Approach
In recent years, the integration of Generative Artificial Intelligence (AI) has sparked a revolution in various industries, offering unparalleled creativity and efficiency. From generating realistic images to composing coherent text, the capabilities of generative AI have shown immense promise. However, along with the potential benefits, businesses must be vigilant about the inherent risks associated with its use.
- Ethical Concerns and Misuse: Generative AI can inadvertently produce content that is offensive, discriminatory, or inappropriate. Businesses deploying this technology need to be cautious about the potential misuse of such content, which could lead to reputation damage, legal consequences, and public backlash. Striking the right balance between creative freedom and ethical boundaries is crucial.
- Intellectual Property and Plagiarism: Generative AI's ability to create content automatically raises concerns about intellectual property rights and plagiarism. If a business unknowingly generates content that closely resembles existing copyrighted material, legal disputes could arise. It's imperative to ensure that the AI's training data and output do not infringe upon intellectual property laws.
- Data Privacy and Security: Utilizing generative AI requires substantial amounts of data, which raises data privacy and security concerns. If not handled properly, sensitive information could be exposed, leading to breaches, legal actions, and damage to consumer trust. Implementing robust security measures and complying with data protection regulations are vital steps in mitigating these risks.
- Unpredictable Outputs: Generative AI's creative process is not always fully controllable, leading to unpredictable outputs. This can be problematic when the generated content doesn't align with the brand's values or messaging. Thorough testing and oversight are necessary to ensure that the AI-generated content consistently reflects the desired tone and message.
- Dependency on Technology: Relying heavily on generative AI can lead to a dangerous over-dependency on technology. Businesses might find themselves in a precarious position if the AI system fails or requires updates. Maintaining human expertise alongside AI implementation can help prevent disruptions to operations.
- Lack of Human Touch: While generative AI can automate and streamline content creation, it might lack the human touch that resonates with audiences. Over-reliance on AI-generated content could lead to a loss of authenticity and connection with customers. Striking a balance between automated and human-created content is essential for maintaining a genuine brand voice.
- Initial Investment and Training: Integrating generative AI into business processes requires a significant upfront investment in terms of technology, training, and infrastructure. If not managed properly, this investment might not yield the expected returns, resulting in financial strain.
To navigate these risks effectively, businesses must adopt a thoughtful and cautious approach to incorporating generative AI:
- Ethical Guidelines: Establish clear ethical guidelines for AI-generated content creation to ensure that the technology is used responsibly and aligns with the company's values.
- Human Oversight: Implement robust human oversight mechanisms to review and edit AI-generated content before publication, ensuring its accuracy and appropriateness.
- Legal Consultation: Seek legal advice to understand the intellectual property and copyright implications of using generative AI, safeguarding against potential legal disputes.
- Data Security: Prioritize data security and privacy by implementing encryption, secure storage, and compliance with relevant data protection regulations.
- Diversification of Content Creation: Maintain a healthy mix of human-generated and AI-generated content to preserve authenticity and avoid over-dependency on technology.
In conclusion, while generative AI offers remarkable capabilities, its deployment comes with a set of business risks that need careful consideration. By adopting a balanced approach, with ethical considerations, human oversight, and a thorough understanding of legal and security implications, businesses can harness the power of generative AI while minimizing potential pitfalls.
So where does this leave us? Unfortunately, for at least the short term, it leaves us with more questions than answers. For better or worse, AI is here to stay, so it is up to us as technology leaders to get in front of the hype cycle and develop not only the skills to manage the technology but to build out our skills to leverage AI as a meaningful business tool as well.
To continue the conversation, I have two challenges for you this month. First, share your experiences with AI with our community—hearing about your successes and failures with AI or any other solutions you are exploring is part of the value of Executive Exchange. Second, take advantage of our upcoming ASUG Executive Exchange Fall Summit to share your experiences in person. September 25–27, we are bringing the ASUG Executive Exchange community together at the W hotel in Nashville for two full days of networking and learning, and we would love to have you at the table. Learn more about the event and register here.